Laying the foundations: Understanding data and its importance
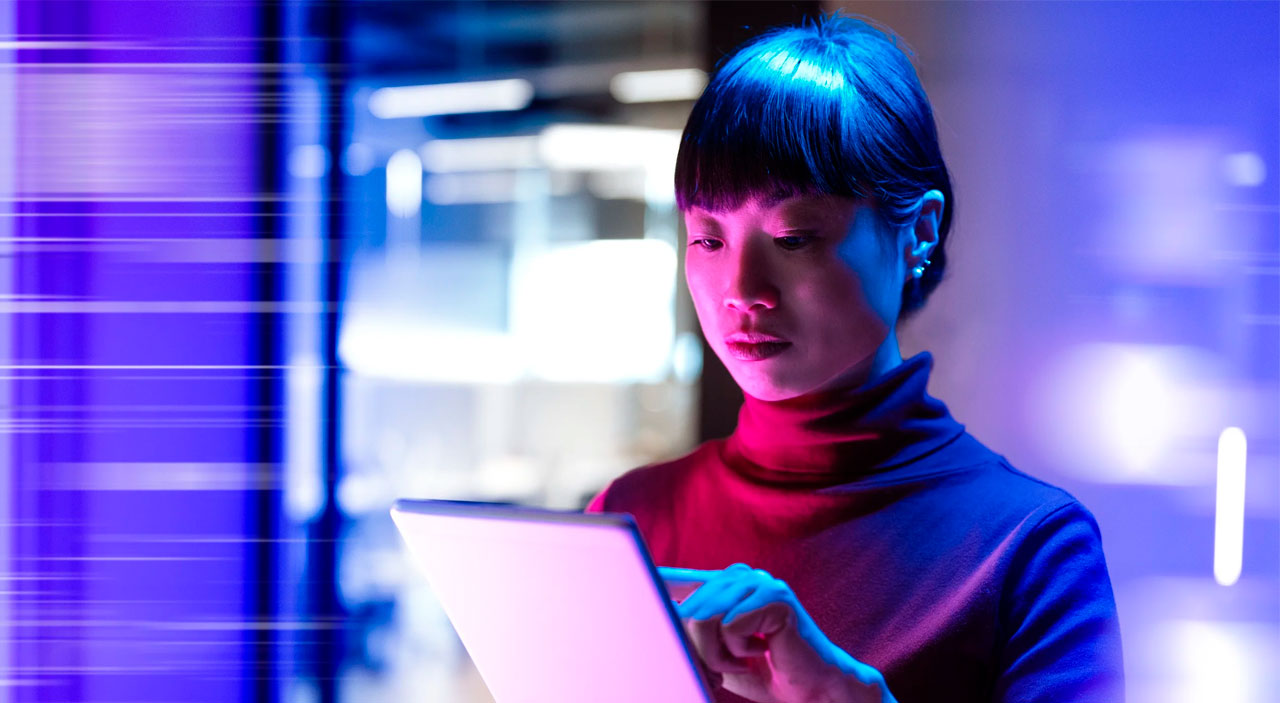
In today's digital world, data is more than just a resource—it is the foundation of business strategy. Often referred to as the new oil, data helps businesses drive efficiencies, make informed decisions, and seize new opportunities.
Still, just like crude oil, raw data must be refined to unlock its real value. Poor data quality, inconsistencies, and fragmentation can hinder an organisation's ability to leverage advanced technologies such as artificial intelligence (AI) and machine learning (ML), ultimately impeding informed decision-making.
This article is the first in a three-part series designed to guide firms through the complexities of data analytics. We begin by examining the fundamentals—defining data analytics, exploring the importance of data quality, and outlining key principles for establishing a strong data-driven foundation.
Data analytics fundamentals: What is it, and why is it important?
Data analytics is the systematic process of gathering, analysing, and interpreting data to uncover trends, patterns, and correlations—transforming raw data into actionable business intelligence. According to Harvard Business School, it spans a range of areas, from descriptive analytics (understanding past events) and diagnostic analytics (identifying causes) to predictive analytics (forecasting future outcomes) and prescriptive analytics (determining optimal courses of action).
Organisations that effectively leverage data analytics gain a competitive edge by improving operational efficiency, identifying new revenue opportunities, strengthening customer relationships, enhancing risk management, and driving AI-powered innovation.
Still, none of these benefits can be achieved without a solid data foundation, starting with data quality.
The importance of high-quality data
Although a powerful asset for driving insights and decision-making, data has limited value if it is not accurate and reliable. Even the most abundant data, if riddled with errors, can lead to suboptimal decisions and missed opportunities.
Poor data quality can distort analyses, misguide decisions, and create operational inefficiencies—sometimes with costly financial and regulatory consequences. In contrast, high-quality data is the backbone of effective data analysis as it equips organisations with insights needed to thrive in an increasingly data-driven world.
How can businesses determine whether their data meets the mark? Here are the key attributes of data quality:
Accuracy: Data accuracy reflects the degree to which data is correct, precise, and without errors. High-quality data is accurate in the sense that it correctly represents real-world values.
Completeness: To be complete, the dataset must contain all necessary records without any redundancy. Missing data or duplicates can skew results and lead to poor decisions.
Consistency: Consistency ensures data values are compatible across different datasets or systems. It is often achieved through data standardisation, which ensures reliability across various systems and reports.
Timeliness: Data holds value only when available in a timely manner. In the current fast-paced business environment, when decisions are often made on the go, stale and obsolete data can lead to missed opportunities or flawed predictions.
Relevance: Data needs to be aligned with business objectives and decision-making needs to ensure that the information focuses on what truly matters.
Investing in data governance, validation processes, and data cleansing tools can help businesses maintain high-quality data that can be used to its full potential.
Practical steps to improve data quality
A strong data foundation is built on a structured approach to data management, which includes the following key steps:
1. Establish data governance frameworks
Implement policies and guidelines to standardise data collection, storage, and usage, ensuring both data integrity and security.
2. Conduct a data audit
Evaluate current data quality, identify missing values or redundancies, and document inconsistencies.
3. Implement data validation and cleansing procedures
Data validation involves the evaluation of datasets for errors, inconsistencies, and inaccuracies, while data cleansing refers to the process of correcting them to improve data quality. Businesses can leverage automation for data cleansing and use automated tools to detect and correct errors, duplicate records, and validate data integrity.
4. Integrate data across systems
Today, businesses often collect and store data in different formats across various points, from databases and spreadsheets to apps and cloud platforms. This fragmentation can lead to data discrepancies. Creating a single source of truth that consolidates data into a centralised, reliable location is essential to maintain consistency and accuracy. This ensures seamless connectivity between key functions such as finance, CRM, and other core business applications, driving alignment across the organisation.
5. Train employees on data best practices
Foster a data-driven culture by educating teams on the importance of data accuracy and governance.
By following these steps organisations can ensure that their data is clean, well-structured, and ready for deeper analytics and AI adoption.
Preparing for advanced technologies
A strong data foundation is a prerequisite for implementing more advanced technologies like AI, machine learning, and process automation, which enable businesses to collect, analyse and—most importantly—act on data at speeds and volumes previously unimaginable.
As data becomes more and more abundant and computing power increases, these technologies allow data analytics to move from historical data analysis towards real-time analytics, allowing businesses to become responsive to market changes at a speed never seen before.
But to be able to adopt and leverage these powerful technologies, businesses must first ensure:
Data is clean, reliable, structured, and well-managed
Reporting and analytics capabilities are in place to derive insights
Employees understand how to interpret and act on data-driven recommendations
Organisations that invest in these areas will be well-positioned to leverage AI for predictive analytics, process automation, and real-time decision-making.
Real-world success story: Turning data into instant action through automated management reporting
To illustrate the transformative power of data analytics, consider the case of a mid-sized rendering company struggling with inefficient manual reporting processes.
The client's CEO dedicated nearly 10 hours each month to extracting incomplete and disorganised data from disparate systems, including their CRM and finance software. This poor data governance left little room for strategic focus and resulted in limited visibility into critical business insights, such as sales trends and customer behaviour. As a result, the company faced difficulties establishing a strong commission structure and optimising customer pricing strategies.
Recognising the need for change, the company teamed up with Hazlewoods to implement a fully automated management reporting platform that automates data extraction and analysis across the client's CRM and finance system. The new system transformed their operations by providing real-time insights, ensuring the data was always accurate and up-to-date.
Customisable dashboards were designed to give a clear view of critical areas such as sales performance, customer behaviour, product performance, and financial metrics. The platform also included automated alerts, which notified the team of key developments like sales target achievement or risks related to customer churn.
One of the most significant results of the new bespoke management reporting system was the improvement in the company's sales strategy.
With a transparent commission structure and real-time sales tracking, employees were more motivated, and retention rates increased. Additionally, enhanced customer intelligence allowed the firm to manage pricing strategies better, optimise loyalty programmes, and improve customer retention.
Real-time insights enabled informed decision-making across key functions, from inventory management to aligning sales strategies with business objectives. Supplier data analysis and streamlined procurement processes resulted in cost-saving measures. Additionally, relationships with customers improved through personalised offers and targeted engagement.
Finally, the company set the stage for future AI-driven analytics and automation, positioning itself for scalable growth.
Building together a data-driven future
Businesses prioritising data analytics not only improve operational efficiency but unlock new opportunities and gain a competitive edge.
The journey to becoming a data-driven organisation begins with a solid foundation and evolves through strategic governance, advanced analytics, and automation. For many businesses, navigating this transformation can be challenging, but with the right expertise, they can harness data as a powerful asset.
HLB's Data Analytics & Business Intelligence group helps organisations navigate the complexities of modern data environments. Our team members with deep expertise in predictive analytics, AI-driven strategies, and scalable data storage solutions help businesses establish a strong data foundation while ensuring robust governance through compliance with security and privacy standards.
Take the next step toward becoming a truly data-driven organisation. Contact our team today to learn how we can help you turn data into a strategic advantage.